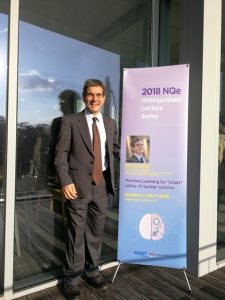
2018 November 13, Enrico Zio gave an Annual Distinguished Lecture at the Korean Atomic Institute of Science and Technology (KAST) with the title: Machine Learning for “smart” safety of nuclear systems.
As the digital, physical and human worlds continue to integrate, the 4th industrial revolution, the internet of things and big data, the industrial internet, are changing the way we design, manufacture, deliver products and services. In this fast-pace changing environment, the attributes related to the reliability of components and the safety of the systems we operate continue to play a fundamental role for industry. On the other hand, the advancements in knowledge, methods and techniques, the increase in information sharing and data availability, offer new ways for reliable and safe engineering of systems and new opportunities of business in several areas of application. Based on this increased knowledge, information and data available, we can improve our prediction capabilities. Particularly, the increased availability of data coming from monitoring the relevant parameters of components, systems and assets performance, and the grown ability of treating these data by intelligent machine learning algorithms, capable of mining out information relevant to the assessment and prediction of their state, has open wide the doors for disruptive advancements in many industrial sectors, for reliable and safe design, operation, management and maintenance.
In this lecture, I frame the different problems that can be tackled by machine learning, both by examples and scientifically, and offer some reflections on the opportunities and challenges related to the use of machine learning in the nuclear industry, focusing in particular on the models, methods, algorithms and tools for modelling complex industrial components and systems and for treating their data, with the objective of detecting, diagnosing and predicting anomalous conditions for anticipating failures and supporting effective, condition-based and predictive risk assessment and maintenance management.